HACID Webinar Series
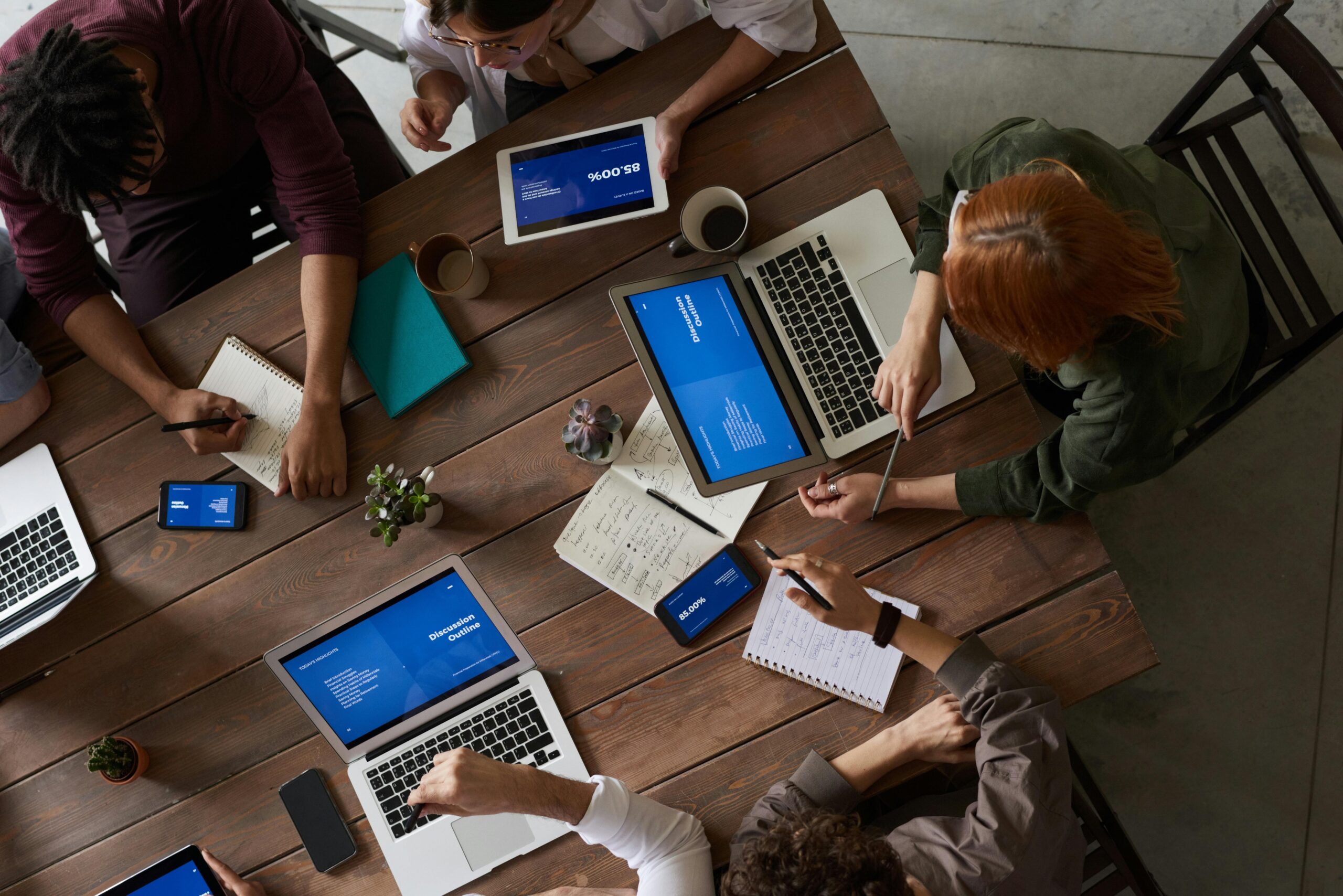
We are organising a series of webinars to dig into the opportunities offered by hybrid collective intelligence, that is, the power of collaboration between humans and machines.
The webinar series is composed of five events, hosted online between February and July 2025:
- Hybrid Collective Intelligence: perspectives and challenges
- Collective Intelligence in the era of LLMs
- Gender and diversity aspects in Hybrid Collective Intelligence
- Hybrid Collective Intelligence for Medical Diagnostics
- Delivering Climate Services at scale through collective intelligence
In the following, we will give details about the webinars as long as they get defined.
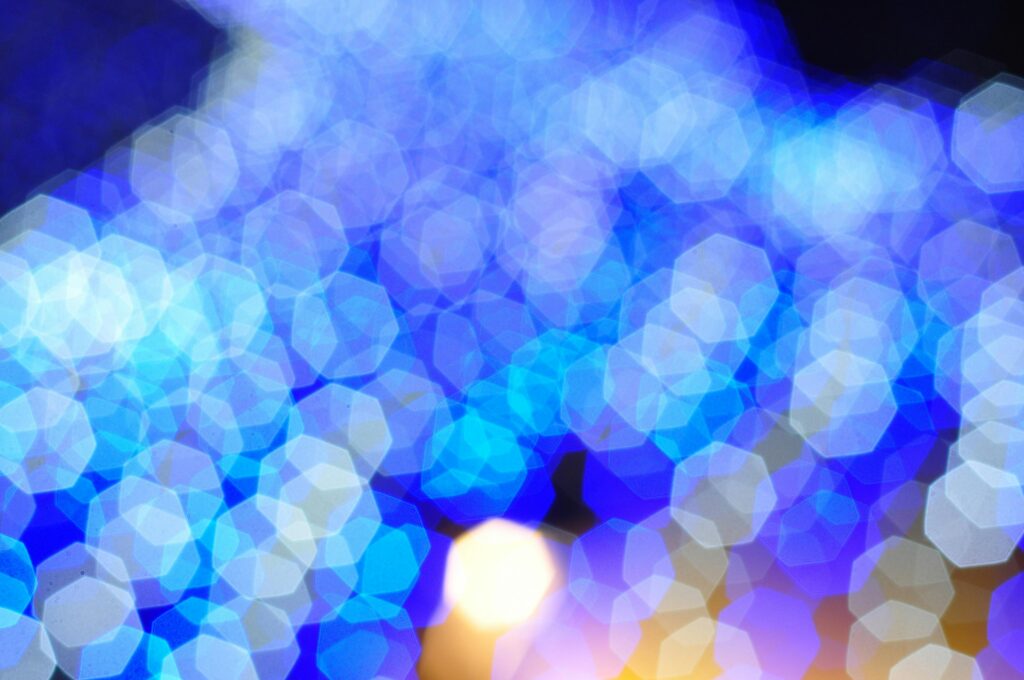
Webinar #1 — Hybrid Collective Intelligence: perspectives and challenges
When: Tuesday, February the 25th at 5pm CET.
Registration required at this link: https://nesta.zoom.us/meeting/register/xEz0RfmeQxag4GZMPPgdXQ
The first webinar will introduce the series with a broad discussion on hybrid collective intelligence. We will discuss perspectives and challenges with renowned experts in the field who will share their latest research before opening up an audience Q&A.
Speakers and talks
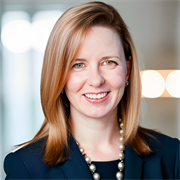
Anita Williams Woolley, Carnegie Mellon University
Dr. Anita Williams Woolley is an expert on teamwork and collective intelligence in human and human-machine collaboration. She is the PI of the CI@CMU lab and a co-I of the NSF AI-CARING Institute. Dr. Woolley just finished a term as Associate Dean of Research at Carnegie Mellon’s Tepper School of Business in July 2024, and prior to that served as the Chair of CMU’s Institutional Review Board from 2019-2022. She is a senior editor at Organization Science, a founding associate editor of the ACM journal Collective Intelligence, and an affiliated faculty member of the MIT Center for Collective Intelligence. Dr. Woolley received her doctorate in organizational behavior from Harvard University, and her research includes seminal work on collective intelligence in teams, first published in Science in 2010 and since discussed and cited in thousands of research publications and news outlets. She and collaborators have built on that work to develop and validate direct and indirect approaches to measuring collective intelligence in a variety of settings, including field experiments in organizations, classroom settings, and in-person and online experimental studies, resulting in dozens of additional publications. Dr. Woolley has been a Principal or co-investigator on a wide range of US federally-funded grants and corporate-sponsored projects, including multi-million dollar efforts by the US Army Research Office among other DoD agencies as well as DARPA, NSF, and the US Department of Homeland Security.
Title: Opportunities for Collective Intelligence in Hybrid Systems: Teaching Algorithms to Detect and Facilitate Good Teamwork
Abstract: The environment surrounding organizations is becoming more complex and dynamic, and the increased use of AI-based technology both contributes to complexity (by enabling us to do more, faster) but can also enhance collective intelligence if designed and integrated effectively. Research on intelligence has focused on the capabilities that enable a system to adapt and accomplish goals in changing environments for over a century. Building on that work, my collaborators and I have examined collective intelligence in human and human-computer systems, focusing on the collective memory, attention, and reasoning functions that need to be fulfilled for intelligence to emerge. In order to enable AI agents to enhance collective intelligence, we need to teach them to differentiate good from bad teamwork. I will describe some of our current research in which we teach AI agents how to recognize the quality of collaboration in human systems and test new ways agents can intervene to increase collective intelligence.
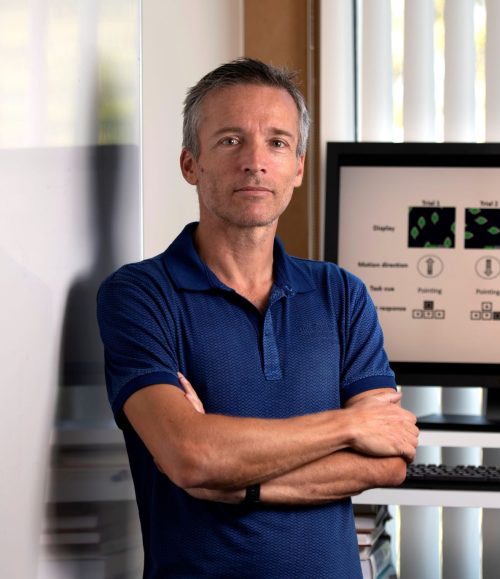
Mark Steyvers, University of California, Irvine
Mark Steyvers is a Professor of Cognitive Science at UC Irvine and Chancellor’s Fellow. He has a joint appointment with the Computer Science department and is affiliated with the Center for Machine Learning and Intelligent Systems. His publications span work in cognitive science as well as machine learning and has been funded by NSF, NIH, IARPA, NAVY, and AFOSR. He received his PhD from Indiana University and was a Postdoctoral Fellow at Stanford University. He is currently serving as Associate Editor of Computational Brain and Behavior and Consulting Editor for Psychological Review and has previously served as the President of the Society of Mathematical Psychology, Associate Editor for Psychonomic Bulletin & Review and the Journal of Mathematical Psychology. In addition, he has served as a consultant for a variety of companies such as eBay, Yahoo, Netflix, Merriam Webster, Rubicon and Gimbal on machine learning problems. Dr. Steyvers received New Investigator Awards from the American Psychological Association as well as the Society of Experimental Psychologists. He also received an award from the Future of Privacy Forum and Alfred P. Sloan Foundation for his collaborative work with Lumosity.
Title: Communicating Uncertainty with LLMs
Abstract: Large language models (LLMs) play a growing role in decision-making, yet their ability to convey and interpret uncertainty remains a challenge. We examine two key issues: (1) how LLMs interpret verbal uncertainty expressions compared to human perception and (2) how discrepancies between LLMs’ internal confidence and their explanations create a disconnect between what users think the model knows and what it actually knows. We identify a calibration gap, where users overestimate LLM accuracy, and a discrimination gap, where explanations fail to help users distinguish correct from incorrect answers. Longer explanations further inflate user confidence without improving accuracy. By aligning LLM explanations with internal confidence, we show that both gaps can be reduced, improving trust calibration and decision-making.
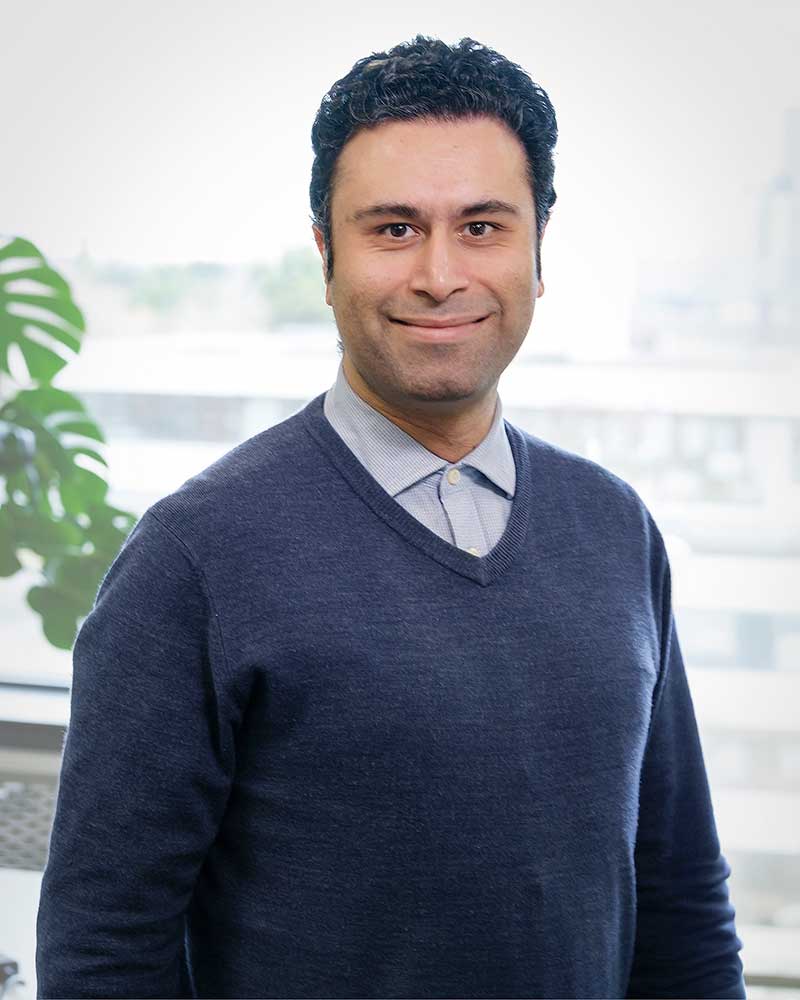
Taha Yasseri, Trinity Collecge Dublin & Technological University of Dublin
Taha Yasseri is the Workday Full Professor and Chair of Technology and Society at Trinity College Dublin and Technological University Dublin. He directs the TCD-TUD Joint Centre for Sociology of Humans and Machines (SOHAM). He is also an adjunct Full Professor at the School of Mathematics and Statistics at University College Dublin.
He was a Professor and the Deputy Head at the School of Sociology and a Geary Fellow at the Geary Institute for Public Policy at University College Dublin, Ireland. Before that, he was a Senior Research Fellow in Computational Social Science at the University of Oxford, a Turing Fellow at the Alan Turing Institute for Data Science and Artificial Intelligence, and a Research Fellow in Humanities and Social Sciences at Wolfson College. Taha Yasseri has a PhD in Complex Systems Physics from the University of Göttingen, Germany. He has interests in analysis of large-scale transactional data and conducting behavioural experiments to understand human dynamics, machines’ social behaviour, government-society interactions, online political behaviour, mass collaboration and collective intelligence, information and opinion dynamics, hate speech and content moderation, collective behaviour, and online dating.
Title: Toward a New Sociology of Humans and Machines: The Dyadic Interactions
Abstract: In the age of hybrid collective intelligence, human-machine interactions are reshaping social dynamics, decision-making, and collaboration. This talk explores how AI enhances and influences collective intelligence, from human-machine social systems to AI-augmented collaboration and the impact of large language models. As an example, I will present findings from human-AI content moderation experiments, illustrating how AI-generated feedback affects user behavior. By examining these dyadic interactions, we can better understand and design resilient, human-centered AI systems.