Webinar #2 — Collective Intelligence in the era of LLMs
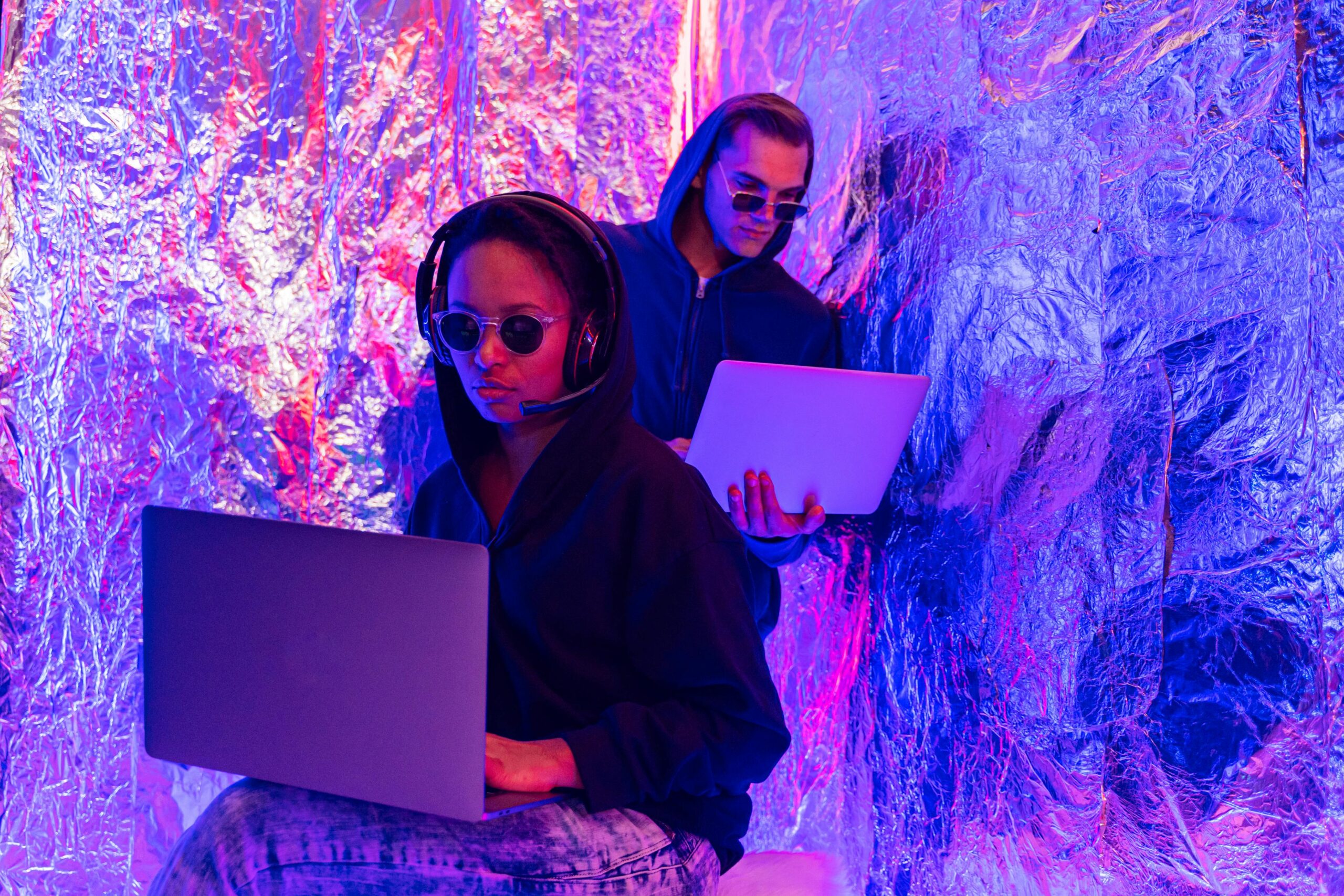
The webinar will explore how LLMs can be employed to support collective intelligence, and how they influence the field at large.
When: Thursday, April the 24th at 5pm CET.
Speakers and talks
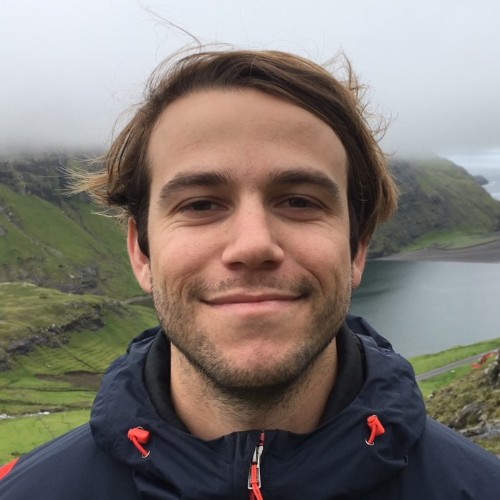
Jason Burton, Copenhagen Business School
Jason Burton is an Assistant Professor at Copenhagen Business School and an Associate Research Scientist at the Max Planck Institute for Human Development. His research examines the mechanisms of human reasoning and decision-making, and how those mechanisms are affected by emerging technologies. His work has been supported by the Nesta Centre for Collective Intelligence Design, the Alexander von Humboldt Foundation, and the Knight First Amendment Institute at Columbia University. He received his PhD in Psychology from Birkbeck, University of London.
Title: How large language models can reshape collective intelligence
Abstract: Collective intelligence underpins the success of groups, organizations, markets, and societies. Through distributed cognition and coordination, collectives can achieve outcomes that exceed the capabilities of individuals—even experts—resulting in improved accuracy and novel capabilities. Often, collective intelligence is supported by information technology, such as online prediction markets that elicit the ‘wisdom of crowds’, online forums that structure collective deliberation, or digital platforms that crowdsource knowledge from the public. Large language models, however, are transforming how information is aggregated, accessed and transmitted online. In this talk, I will highlight some key ways in which this transformation is reshaping collective intelligence writ large.
See also Burton et al. (2024). How large language models can reshape collective intelligence. Nature Human Behaviour, 8(9), 1643–1655. https://doi.org/10.1038/s41562-024-01959-9
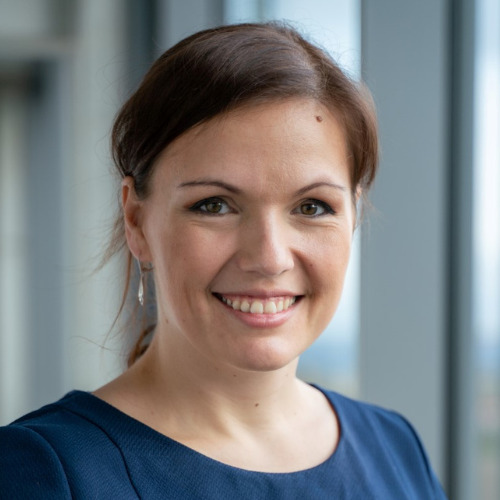
Lucie Flek, University of Bonn
Lucie Flek is a full professor at the University of Bonn, leading the Data Science and Language Technologies group. Her main interests lie in machine learning research for natural language processing (NLP), including AI robustness and safety. The application areas range from large language models and conversational systems, across clinical NLP and mental health research, to misinformation detection and social media analyses. Prof. Flek has been active both in academia and industry – she used to manage natural language understanding research programs in Amazon Alexa and contributed to the Google Shopping Search launch in Europe. Her academic work at the University of Pennsylvania and University College London revolved around user modeling from text, and its applications in psychology and social sciences. Her PhD at TU Darmstadt focused on meaning ambiguity, incorporating expert lexical-semantic resources into DNN classification tasks. She has served as Area Chair for Computational Social Sciences at numerous ACL* conferences, and as an editor of the NLP section of multiple AI journals. Before her career path in natural language processing, Prof. Flek has been contributing to particle physics research at CERN in the area of axion searches.
Title – Pluralistic LLMs: Challenges in modeling and evaluation of perspective taking
Abstract: As LLMs become increasingly powerful, it is critical that they robustly and transparently reflect the complex human values and the broad spectrum of individual views and preferences. Not only the users expect the models to be factually reliable, but also to represent diverse societal perspectives and individual needs. But how, when and what can and shall the LLMs represent? Addressing this modeling challenge is essential for ensuring the models are safe, beneficial, and equitable as they become more deeply integrated into our personal and professional lives.
The talk is based on our recent papers:
– https://aclanthology.org/2024.findings-acl.387/ (ACL 2024)
– https://arxiv.org/abs/2503.13621 (ICLR BiAlign 2025)
– https://aclanthology.org/2024.c3nlp-1.6/ (ACL C3NLP 2024)
– https://aclanthology.org/2022.emnlp-main.500/ (EMNLP 2022)
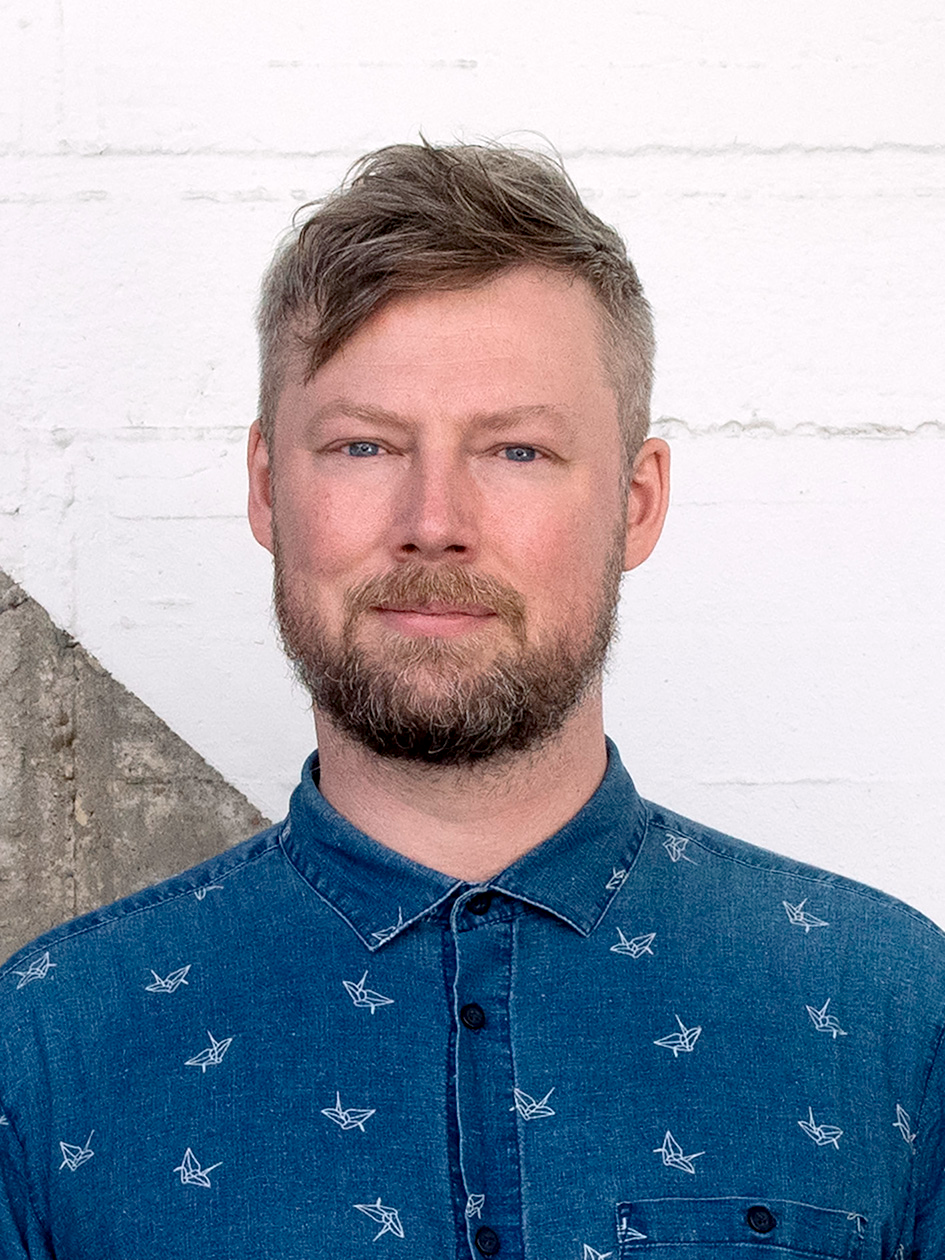
Nikolas Zöller, Max Plank Institute for Human Development
Nikolas Zöller is a postdoctoral researcher at the Center for Adaptive Rationality at the Max Planck Institute for Human Development in Berlin. He employs computational social science methods—such as network analysis, machine learning, and agent-based modeling—to explore how emerging technologies, including artificial intelligence, shape collective decision-making. Before joining the Max Planck Institute, he served as a Research Associate at the Institute for Urban Futures in Potsdam. He completed his PhD in Data Engineering at Constructor University Bremen, where he investigated the dynamics of collaborative groups in open source software communities. Nikolas also worked as a freelance data scientist and software developer, creating data-driven dashboards, interactive visualizations, and machine learning models for clients in media and the arts.
Title: Enhancing medical diagnostics with hybrid collective intelligence
Abstract: Artificial intelligence systems, particularly large language models (LLMs), are increasingly employed in high-stakes decision-making with significant societal impact—yet often without sufficient safeguards for safety, quality, or equity. LLMs can hallucinate, lack common sense, and exhibit bias, issues that may reflect fundamental limitations not easily resolved through more data, more advanced architectures, or additional human feedback. Consequently, relying on LLMs alone for critical, complex tasks remains problematic. This talk introduces a hybrid collective intelligence system designed to overcome these constraints by leveraging the complementary strengths of human expertise and the extensive knowledge captured by LLMs. We tested this approach in open-ended medical diagnostics by combining over 40,000 differential diagnoses from physicians with the diagnoses of five state-of-the-art LLMs across more than 2,000 text-based medical case vignettes. Our findings reveal that physician–LLM collectives outperform not only individual physicians and physician-only collectives, but also individual LLMs and LLM ensembles. This enhanced diagnostic accuracy holds across various medical specialties and levels of professional experience, demonstrating that humans and LLMs make different kinds of errors and thus benefit from each other’s complementary contributions.