🚀 New Preprint from the HACID Project!
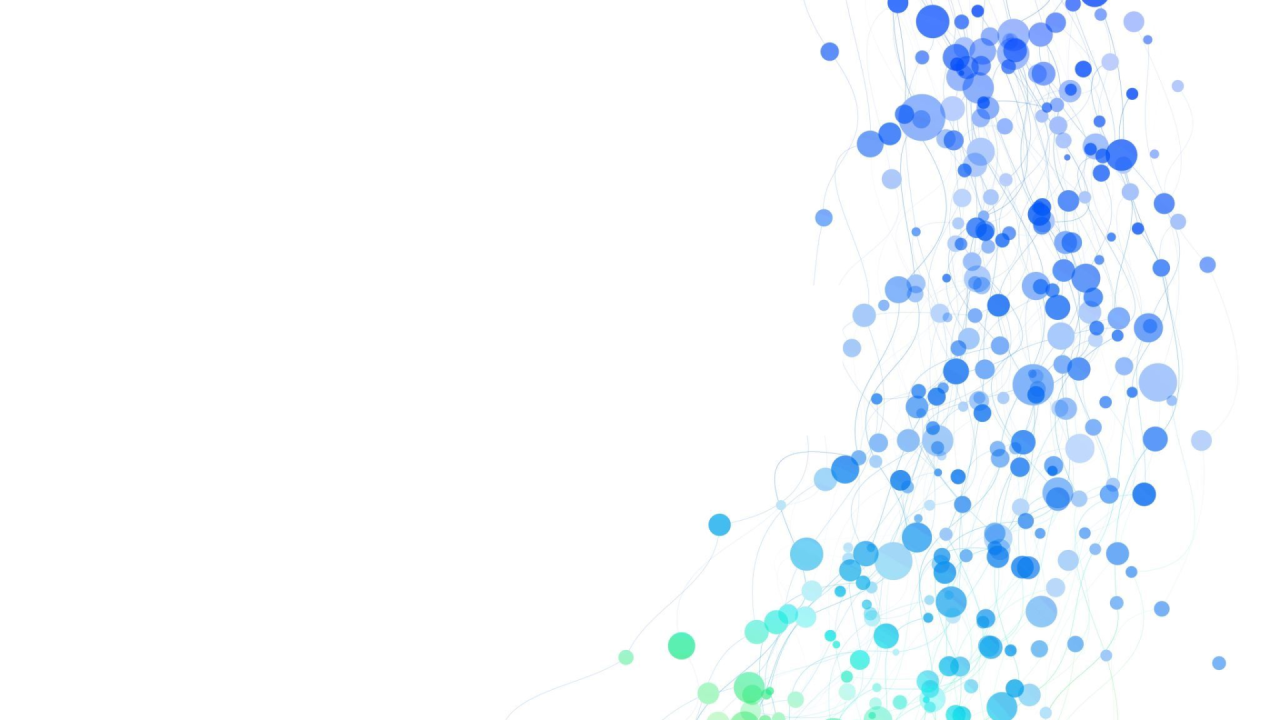
25 June 2024
We are excited to share our latest findings in the preprint “Human-AI collectives produce the most accurate differential diagnoses.” This study demonstrates that human-AI collectives, combining human expertise with advanced AI models, significantly improve diagnostic accuracy.
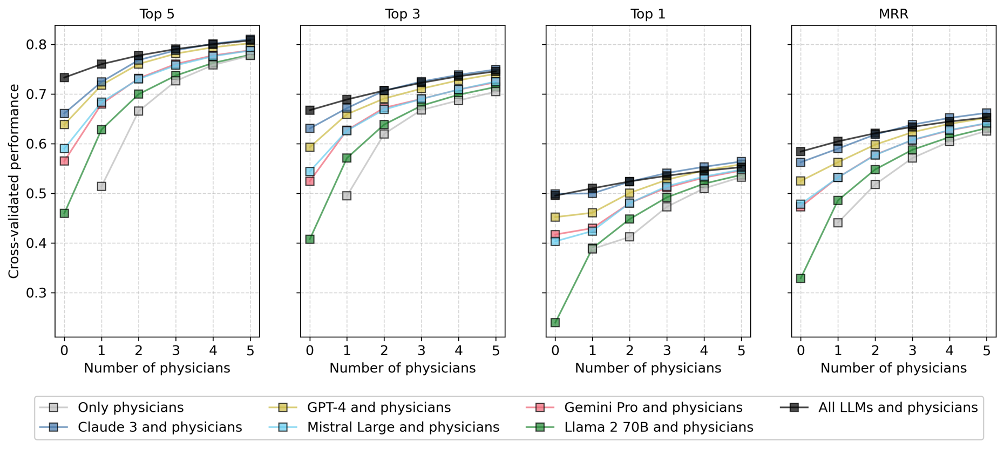
Key Highlights:
- We analyzed 2,133 medical cases and 40,762 physician diagnoses from the Human Diagnosis Project to compare human-only, AI-only, and hybrid collectives. The combination of AI and physician expertise produces superior outcomes. 🤝🤖🧠
- Our findings reveal that humans and AI make different types of errors, and their complementary strengths lead to higher diagnostic accuracy. When AI misses a diagnosis, humans often get it right, and vice versa. This synergy is crucial for better performance. 🩺❌💡⚖️
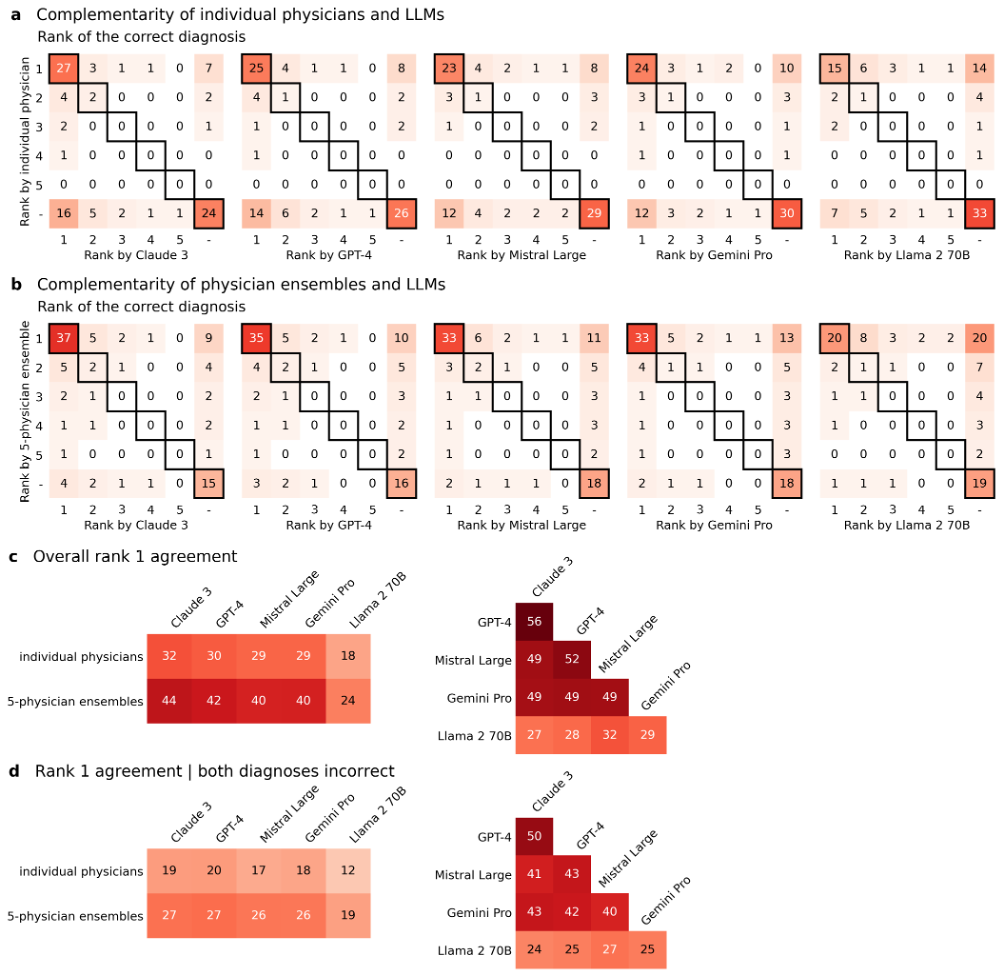
- We utilized state-of-the-art large language models, including Anthropic Claude 3 Opus, Google Gemini Pro 1.0, Meta Llama 2 70B, Mistral Large, and OpenAI GPT-4, to diagnose the same medical cases as human doctors, aggregating their responses into collective diagnoses. 🤖🧠🩺
- Medical specialties such as cardiology, gastroenterology, and infectious diseases all benefited from this hybrid approach, highlighting the broad applicability and potential for improving diagnostic accuracy across various medical fields. 📚
- Using SNOMED CT healthcare terminology and advanced NLP techniques, we automatically harmonized and aggregated diagnoses from both humans and AI, eliminating the need for human intervention in this step. 🛠️🔄
- Diagnostic errors cause nearly 795,000 deaths and permanent disabilities annually in the U.S. alone. Our approach aims to reduce these errors and improve patient outcomes without significantly increasing costs. 🌍📈
- We used case vignettes in text form for this study. Future research could explore integrating multimodal data and assessing performance in real-world clinical settings and across diverse populations, while addressing potential biases. 🔮
Read the full preprint here: http://arxiv.org/abs/2406.14981